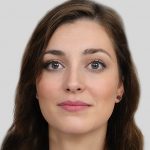
Anna Shalomova
PPM Consultant
PPM and Power BI enables improved overview and better decision-making, more efficient collaboration, reduced maintenance cost, and a customized solution for a clear overview of financials, resources, and portfolio health, allowing for portfolio forecasting. Portfolio Forecasting is the ability to anticipate the impact of economic and market changes on your portfolio. You can build loss forecasts if you guess the future economic conditions right. Portfolio Forecasting extends the concept of forecasting to directly impact revenues Power BI is filled with many exciting features, including the newest analytics feature, clustering. Power View in Power BI gives users advanced statistical analysis tools. It empowers you to generate forecasts that incorporate trends and seasonal factors. It also has table and matrix features. Forecasting is available both on Power BI Desktop and the Power BI online. You can see your forecasting in reports and create new forecasts, not just in the Desktop version.
Portfolio forecasting, as you know, requires vast amounts of historical data. Power BI by itself, cannot store so much data. So, when using it to “predict the future,” you might need to equip yourself with some storage for data. You can go either set up a database or use FluentPro DataMart Cloud. In the second case, the data can be constantly streamed to the Datamart, then be “pulled” to Power BI for forecasting.
Forecasting in Power View is based on a proven effective model for prediction called “exponential smoothing.” More than one method has been developed for the analysis of time series. They differ depending on whether the data is strongly seasonal or has no seasonality, contains “surprises” or irregular peaks, and even how much “noise” you’ve got in your data. “Exponential smoothing” has an excellent track record in academia and business. It has the advantage of suppressing noise and “surprise” variation that can distort the model. But it still allows you to keep up with the trends.
Four basic steps to get started with forecasting
Power BI can predict the future costs of our projects! To do that, you have to switch to the analytics pane of the visual and apply forecasting underneath the “Forecast” option. Power BI can extrapolate the input data into what could be the possible project costs based on the parameters configured. Several visual parameters within Power BI require simple configuration. They are:
- Forecast Length: This describes the number of points or the duration of time that Power BI predicts data for. This can be either of Year(s), Month(s), Day(s), etc.
- Ignore Last: This allows the user to ignore a few points within the dataset. For example, if the dataset uses project costs for the past 12 months and we know that the costs for the last 2 months are incorrect and shouldn’t be included in to forecast, you can do that by ignoring Last.
- Confidence Interval: This option allows us to set the confidence interval, which determines the probability of an absolute value being close to the predicted value, i.e., there is a 95% chance of the actual value being within the range of the expected value.
- Seasonality: Datasets exhibit seasonality when the time value in the dataset exhibits a pattern. Using this option, we can specify seasonality in the given dataset, whether yearly, quarterly or monthly.
The version of the algorithm that considers seasonal data is called “ETS AAA, ” also known as the Holt-Winters algorithm. The one for non-seasonal data is “ETS AAN.” Power View uses the appropriate model automatically based on an analysis of the historical data. The seasonal algorithm uses an equation that accounts for additive error, additive trend, and additive seasonality. It is widely used, for example, in predicting and planning demand in businesses. In Power BI, the algorithm is an enhancement to be resistant to noise in the data. Specifically:
- Use of validation window for optimal parameter selection
- State vector correction at the end of the training window when data is noisy
The non-seasonal algorithm (ETS AAN) uses a simpler equation. It does not consider seasonality at all.
How does the seasonality value affect forecasts?
Seasonality differs by the number of time frames in one complete data cycle before t repeats itself throughout the time series. For example, if you have sales data that changes over a year but tends to look the same year over year, then the time series has a periodicity of one year. If your historical data is presented in units of months, and of course, 12 months comprise a year, then to get the best results, you would set a seasonality value of 12, meaning 12 units makes one complete data cycle. Seasonality estimation strongly affects time series forecasts and is an essential first step when predicting just about everything cyclical in your portfolio’s future. Popular time series forecasting algorithms are:
- Exponential Time Smoothing
- Auto-Regressive Integrated Moving Average
They both require seasonality as input and are often very sensitive to different input values.
Power BI can forecast the future of your data using built-in predictive analytics. In this day and age of an ever-changing business insights landscape, simply generating insights from current/past project data to track project cost isn’t always enough – project managers and business users within your organization need to forecast future project costs so that they can take corrective action today and gain a competitive advantage tomorrow.
Get help with Power BI
by scheduling a free consultation with FluentPro Team